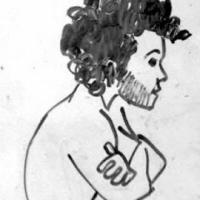
Research interests
I am interested in the non-equilibrium dynamics of many-body quantum systems. I worked with both non-interacting and interacting (integrable or non-integrable) models. I have considerable expertise with numerical tools based on Tensor Network description of many-body quantum states: I dealt with developing MPS (for closed systems) and MPDO (for open system) with special attention to symmetries implementation and parallel optimization. Recently, I started to investigate how MPS, PEPS or Tree Tensor Networks may be proficiently applied in combination with Machine Learning techniques.
I am also an expert on a number of advanced toopics such as noninteracting quantum models, Thermodynamic Bethe Ansatz, entanglement entropies and quasi-particle picture. I have been always interested in developing new analytical and numerical methods or reformulating older ones in a more suitable way. This is important for the success of any project, which faces the limitations of the current analytical and numerical techniques.
I made several key contributions to these exciting areas of research and some of my works have become standard references: I developed a new framework for strongly interacting bosons to calculate the exact dynamics of correlation functions. I applied a semiclassical approach to characterise the dynamical confinement emerging in non-integrable spin chains. I implemented and enriched the numerical algorithms based on Matrix Product States in order to obtain an efficient description of quantum probability distribution functions of local observables. Recently, I developed (in collaboration with people from abroad) what is now known as “generalised hydrodynamics” (GHD): starting from a very simple physical picture, we have derived a continuity equation describing the late-time dynamics of inhomogeneous states in interacting integrable models.
Ongoing research:
- Developing Tensor Network in different representations: momentum/real-space mixing; as well encoding the TDVP in such framework.
- Full Counting Statistics out-of-equilibrium: inspecting time-dependent phase of matter; long-range interactions for long-range order at finite energy density.
- Tensor Network and Machine Learning: e.g. for quantum entanglement (or quantum magic) recognition.
- Measurement-induced quantum phase transition: from Lindbladian to quantum-trajectories.
- Emerging confinement effects in quantum systems: from spin chains to LGTs; suppression of transport; disorder-free many-body localisation.
- Clifford circuits and non-stabiliserness. Tensor-Network techniques tailored to deal with quantum magic resource.